TMARKER
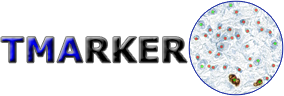
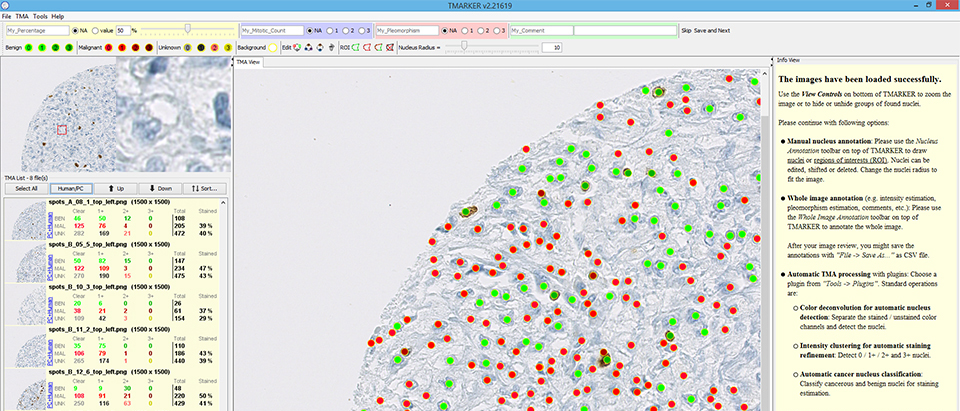
TMARKER assists in cell nuclei counting and staining estimation of pathological immunohistochemical TMA images and non-TMA images.
Two main scenarios are addressed with TMARKER:
- You want to know how many cell nuclei on the image are positive and negative for a certain protein. Therefore, you already did an immunohistochemical staining experiment. TMARKER provides reproducable, stable and accurate cell counting and staining estimation assistance with color deconvolution.
- For staining estimation, you only want to consider one type of cells in the image (e.g. only relevant cancer cells). TMARKER provides modern machine learning algorithms that detect these relevant cells in the image and perform staining estimation only on these cells. Also this procedure is reproducible, stable and transferable.
Download the OS-agnostic Java distribution here.
Academic Partners:
- ETH Zurich, Switzerland,
- NEXUS Personalized Health Technologies, Switzerland
- University Spital Zurich, Switzerland
- Thomas Fuchs Lab, New York, USA
Please cite TMARKER as you use it:
Peter J. Schüffler, Thomas J. Fuchs, Cheng S. Ong, Peter Wild and Joachim M. Buhmann.
TMARKER: A Free Software Toolkit for Histopathological Cell Counting and Staining Estimation.
Journal of Pathology Informatics, vol. 4, 2, p. 2, 2013 doi: 10.4103/2153-3539.109804
PDF URL BibTeX Endnote / RIS Abstract
Download BibTeX citation
@@article{schuffler_tmarker_2013,
title = {{TMARKER}: {A} {Free} {Software} {Toolkit} for {Histopathological} {Cell} {Counting} and {Staining} {Estimation}},
volume = {4},
url = {https://www.sciencedirect.com/science/article/pii/S2153353922006629?via%3Dihub},
doi = {10.4103/2153-3539.109804},
abstract = {Background: Histological tissue analysis often involves manual cell counting and staining estimation of cancerous cells. These assessments are extremely time consuming, highly subjective and prone to error, since immunohistochemically stained cancer tissues usually show high variability in cell sizes, morphological structures and staining quality. To facilitate reproducible analysis in clinical practice as well as for cancer research, objective computer assisted staining estimation is highly desirable. Methods: We employ machine learning algorithms as randomized decision trees and support vector machines for nucleus detection and classification. Superpixels as segmentation over the tissue image are classified into foreground and background and thereafter into malignant and benign, learning from the user's feedback. As a fast alternative without nucleus classification, the existing color deconvolution method is incorporated. Results: Our program TMARKER connects already available workflows for computational pathology and immunohistochemical tissue rating with modern active learning algorithms from machine learning and computer vision. On a test dataset of human renal clear cell carcinoma and prostate carcinoma, the performance of the used algorithms is equivalent to two independent pathologists for nucleus detection and classification. Conclusion: We present a novel, free and operating system independent software package for computational cell counting and staining estimation, supporting IHC stained tissue analysis in clinic and for research. Proprietary toolboxes for similar tasks are expensive, bound to specific commercial hardware (e.g. a microscope) and mostly not quantitatively validated in terms of performance and reproducibility. We are confident that the presented software package will proof valuable for the scientific community and we anticipate a broader application domain due to the possibility to interactively learn models for new image types.},
number = {2},
journal = {Journal of Pathology Informatics},
author = {Sch\"uffler, Peter J. and Fuchs, Thomas J. and Ong, Cheng S. and Wild, Peter and Buhmann, Joachim M.},
year = {2013},
pages = {2},
}
title = {{TMARKER}: {A} {Free} {Software} {Toolkit} for {Histopathological} {Cell} {Counting} and {Staining} {Estimation}},
volume = {4},
url = {https://www.sciencedirect.com/science/article/pii/S2153353922006629?via%3Dihub},
doi = {10.4103/2153-3539.109804},
abstract = {Background: Histological tissue analysis often involves manual cell counting and staining estimation of cancerous cells. These assessments are extremely time consuming, highly subjective and prone to error, since immunohistochemically stained cancer tissues usually show high variability in cell sizes, morphological structures and staining quality. To facilitate reproducible analysis in clinical practice as well as for cancer research, objective computer assisted staining estimation is highly desirable. Methods: We employ machine learning algorithms as randomized decision trees and support vector machines for nucleus detection and classification. Superpixels as segmentation over the tissue image are classified into foreground and background and thereafter into malignant and benign, learning from the user's feedback. As a fast alternative without nucleus classification, the existing color deconvolution method is incorporated. Results: Our program TMARKER connects already available workflows for computational pathology and immunohistochemical tissue rating with modern active learning algorithms from machine learning and computer vision. On a test dataset of human renal clear cell carcinoma and prostate carcinoma, the performance of the used algorithms is equivalent to two independent pathologists for nucleus detection and classification. Conclusion: We present a novel, free and operating system independent software package for computational cell counting and staining estimation, supporting IHC stained tissue analysis in clinic and for research. Proprietary toolboxes for similar tasks are expensive, bound to specific commercial hardware (e.g. a microscope) and mostly not quantitatively validated in terms of performance and reproducibility. We are confident that the presented software package will proof valuable for the scientific community and we anticipate a broader application domain due to the possibility to interactively learn models for new image types.},
number = {2},
journal = {Journal of Pathology Informatics},
author = {Sch\"uffler, Peter J. and Fuchs, Thomas J. and Ong, Cheng S. and Wild, Peter and Buhmann, Joachim M.},
year = {2013},
pages = {2},
}
Download Endnote/RIS citation
TY - JOUR
TI - TMARKER: A Free Software Toolkit for Histopathological Cell Counting and Staining Estimation
AU - Schüffler, Peter J.
AU - Fuchs, Thomas J.
AU - Ong, Cheng S.
AU - Wild, Peter
AU - Buhmann, Joachim M.
T2 - Journal of Pathology Informatics
AB - Background: Histological tissue analysis often involves manual cell counting and staining estimation of cancerous cells. These assessments are extremely time consuming, highly subjective and prone to error, since immunohistochemically stained cancer tissues usually show high variability in cell sizes, morphological structures and staining quality. To facilitate reproducible analysis in clinical practice as well as for cancer research, objective computer assisted staining estimation is highly desirable. Methods: We employ machine learning algorithms as randomized decision trees and support vector machines for nucleus detection and classification. Superpixels as segmentation over the tissue image are classified into foreground and background and thereafter into malignant and benign, learning from the user's feedback. As a fast alternative without nucleus classification, the existing color deconvolution method is incorporated. Results: Our program TMARKER connects already available workflows for computational pathology and immunohistochemical tissue rating with modern active learning algorithms from machine learning and computer vision. On a test dataset of human renal clear cell carcinoma and prostate carcinoma, the performance of the used algorithms is equivalent to two independent pathologists for nucleus detection and classification. Conclusion: We present a novel, free and operating system independent software package for computational cell counting and staining estimation, supporting IHC stained tissue analysis in clinic and for research. Proprietary toolboxes for similar tasks are expensive, bound to specific commercial hardware (e.g. a microscope) and mostly not quantitatively validated in terms of performance and reproducibility. We are confident that the presented software package will proof valuable for the scientific community and we anticipate a broader application domain due to the possibility to interactively learn models for new image types.
DA - 2013///
PY - 2013
DO - 10.4103/2153-3539.109804
VL - 4
IS - 2
SP - 2
UR - https://www.sciencedirect.com/science/article/pii/S2153353922006629?via%3Dihub
ER -
TI - TMARKER: A Free Software Toolkit for Histopathological Cell Counting and Staining Estimation
AU - Schüffler, Peter J.
AU - Fuchs, Thomas J.
AU - Ong, Cheng S.
AU - Wild, Peter
AU - Buhmann, Joachim M.
T2 - Journal of Pathology Informatics
AB - Background: Histological tissue analysis often involves manual cell counting and staining estimation of cancerous cells. These assessments are extremely time consuming, highly subjective and prone to error, since immunohistochemically stained cancer tissues usually show high variability in cell sizes, morphological structures and staining quality. To facilitate reproducible analysis in clinical practice as well as for cancer research, objective computer assisted staining estimation is highly desirable. Methods: We employ machine learning algorithms as randomized decision trees and support vector machines for nucleus detection and classification. Superpixels as segmentation over the tissue image are classified into foreground and background and thereafter into malignant and benign, learning from the user's feedback. As a fast alternative without nucleus classification, the existing color deconvolution method is incorporated. Results: Our program TMARKER connects already available workflows for computational pathology and immunohistochemical tissue rating with modern active learning algorithms from machine learning and computer vision. On a test dataset of human renal clear cell carcinoma and prostate carcinoma, the performance of the used algorithms is equivalent to two independent pathologists for nucleus detection and classification. Conclusion: We present a novel, free and operating system independent software package for computational cell counting and staining estimation, supporting IHC stained tissue analysis in clinic and for research. Proprietary toolboxes for similar tasks are expensive, bound to specific commercial hardware (e.g. a microscope) and mostly not quantitatively validated in terms of performance and reproducibility. We are confident that the presented software package will proof valuable for the scientific community and we anticipate a broader application domain due to the possibility to interactively learn models for new image types.
DA - 2013///
PY - 2013
DO - 10.4103/2153-3539.109804
VL - 4
IS - 2
SP - 2
UR - https://www.sciencedirect.com/science/article/pii/S2153353922006629?via%3Dihub
ER -
Background: Histological tissue analysis often involves manual cell counting and staining estimation of cancerous cells. These assessments are extremely time consuming, highly subjective and prone to error, since immunohistochemically stained cancer tissues usually show high variability in cell sizes, morphological structures and staining quality. To facilitate reproducible analysis in clinical practice as well as for cancer research, objective computer assisted staining estimation is highly desirable. Methods: We employ machine learning algorithms as randomized decision trees and support vector machines for nucleus detection and classification. Superpixels as segmentation over the tissue image are classified into foreground and background and thereafter into malignant and benign, learning from the user's feedback. As a fast alternative without nucleus classification, the existing color deconvolution method is incorporated. Results: Our program TMARKER connects already available workflows for computational pathology and immunohistochemical tissue rating with modern active learning algorithms from machine learning and computer vision. On a test dataset of human renal clear cell carcinoma and prostate carcinoma, the performance of the used algorithms is equivalent to two independent pathologists for nucleus detection and classification. Conclusion: We present a novel, free and operating system independent software package for computational cell counting and staining estimation, supporting IHC stained tissue analysis in clinic and for research. Proprietary toolboxes for similar tasks are expensive, bound to specific commercial hardware (e.g. a microscope) and mostly not quantitatively validated in terms of performance and reproducibility. We are confident that the presented software package will proof valuable for the scientific community and we anticipate a broader application domain due to the possibility to interactively learn models for new image types.